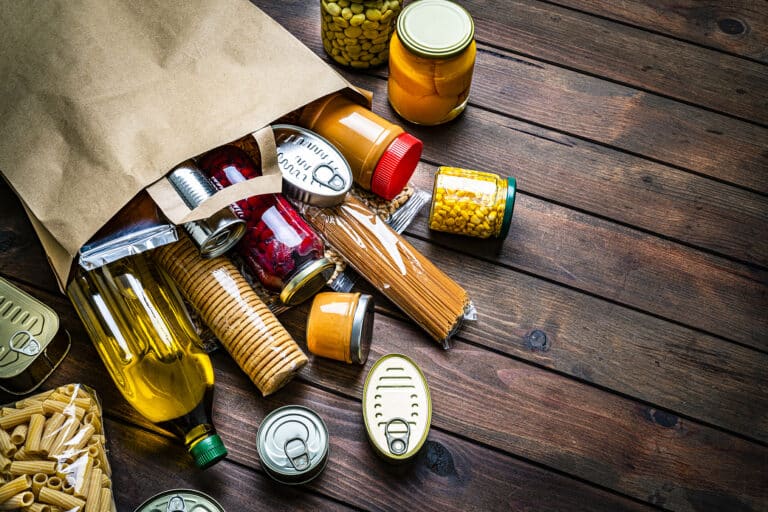
The Future of Packaging: Balancing Sustainability, Cost, and Innovation
We will explore how businesses can more effectively navigate an evolving market landscape by critically evaluating their existing packaging strategies
and exploring innovative alternatives.